Abstract
Automatic large-scale mapping of land cover classes facilitates applications in sustainable development, agriculture, and urban planning, and is therefore a commonly studied topic in remote sensing image processing, but typical deep learning approaches use models pre-trained on everyday image datasets like ImageNet and retrain them using only three channels (usually RGB), not fully leveraging the unique properties and spectral information of multispectral satellite images.
The latest approach in our series of approaches, powered by new open satellite imagery datasets like BigEarthNet and machine learning libraries like fast.ai and eo-learn, explores the effect of pre-training convolutional neural networks on multispectral satellite imagery for country-scale land cover predictions on data for all of Slovenia in 2017.
“Deep Transfer Learning for Land Cover Classification on Open Multispectral Satellite Imagery”: video, slides
Presented at FOSS4G 2019
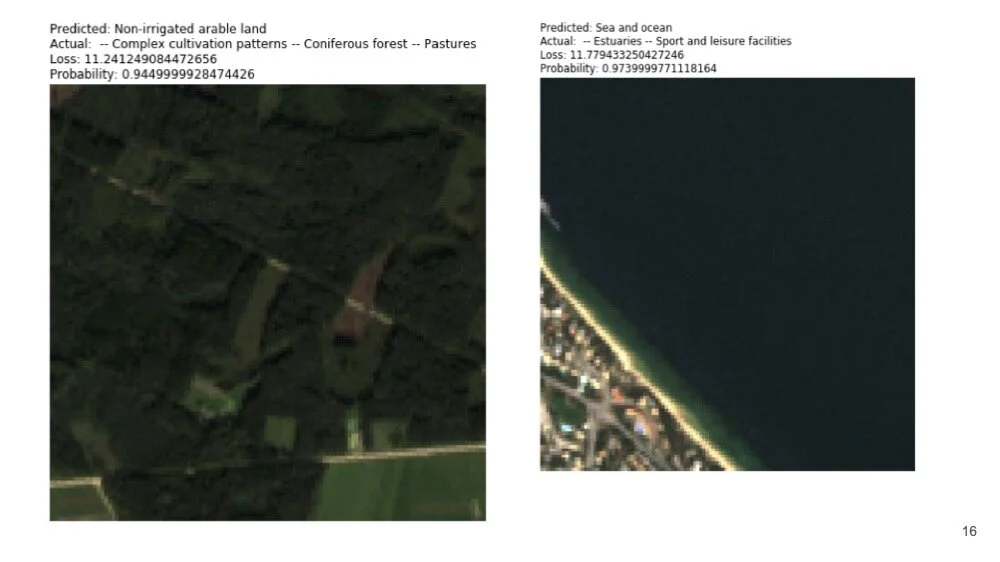
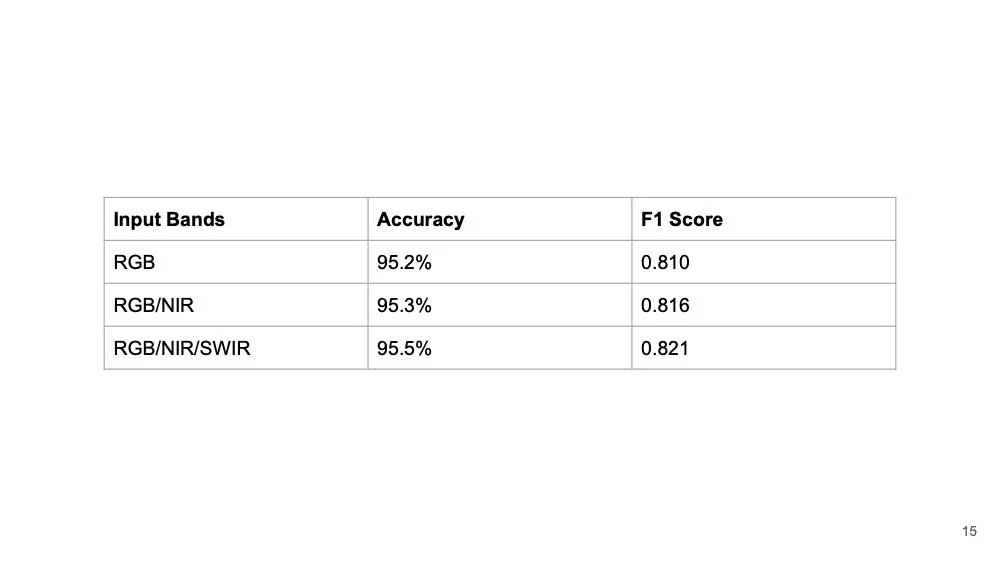
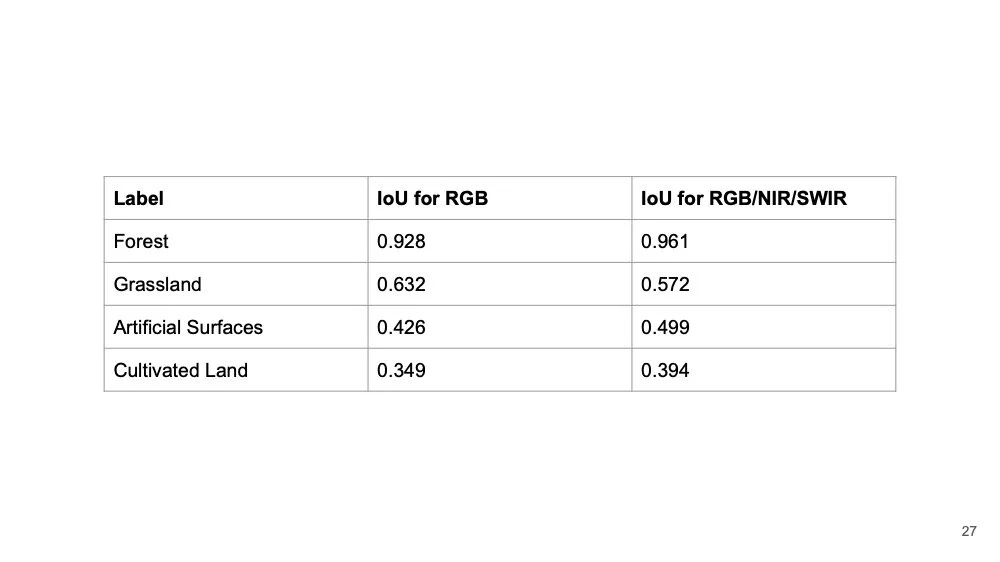